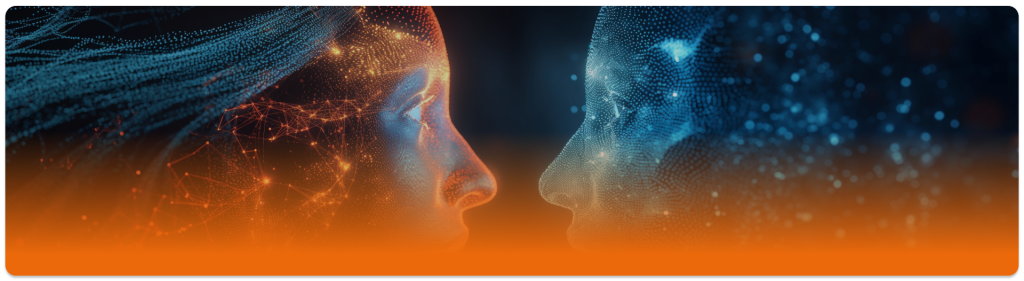
In today’s fast-paced landscape, artificial intelligence (AI) stands at the forefront of innovation. Understanding the nuances of AI trends, particularly predictive AI and generative AI, is crucial for grasping the trajectory of future developments.
Artificial intelligence (AI) has evolved from theoretical concepts to practical applications in multiple industries, experiencing exponential growth through the transition from rule-based systems to machine learning and deep learning algorithms. As AI becomes integral to modern society, understanding its ongoing trends is crucial for driving technological advancement and societal progress, revolutionizing business processes and enhancing healthcare outcomes.
Predictive AI: A Deep Dive
Predictive AI encompasses algorithms and models designed to forecast future outcomes based on historical data patterns. By leveraging advanced statistical techniques and machine learning algorithms, predictive AI empowers organizations to make informed decisions and anticipate future trends.
Predictive AI finds applications across diverse industries, including finance, healthcare, marketing, and manufacturing. From predicting customer behavior to optimizing supply chain logistics, the applications of predictive AI are vast and multifaceted.
Advantages of Predictive AI Systems
Predictive AI equips organizations with the ability to make data-driven decisions, thereby minimizing risks and maximizing opportunities. By analyzing large datasets and identifying patterns, predictive AI enables proactive decision-making in dynamic business environments.
In industries such as retail and e-commerce, predictive AI enhances the customer experience by personalizing recommendations and anticipating consumer preferences. By understanding individual behaviors and preferences, businesses can tailor their offerings to meet the unique needs of each customer.
In the manufacturing sector, predictive AI plays a crucial role in optimizing equipment maintenance schedules and minimizing downtime. By analyzing sensor data and identifying potential failures in advance, predictive AI enables proactive maintenance strategies, thereby reducing costs and improving operational efficiency.
Challenges and Limitations of Predictive AI
One of the primary challenges associated with predictive AI is the ethical and legal implications surrounding data privacy. As organizations collect and analyze vast amounts of personal data, concerns arise regarding the potential misuse or unauthorized access to sensitive information.
Predictive AI algorithms rely heavily on historical data patterns to make future predictions. However, this reliance on past data may lead to biases and inaccuracies, particularly in rapidly evolving environments where historical trends may not accurately reflect future outcomes.
Generative AI: A Deep Dive
Generative AI, also known as creative AI, refers to algorithms capable of generating new content, such as images, music, or text, that mimics human creativity. By employing techniques such as deep learning and neural networks, generative AI unlocks new possibilities in creative expression and innovation.
Generative AI finds applications across a wide range of creative industries, including art, music, fashion, and design. From generating unique artworks to composing original music, generative AI empowers artists and creators to explore new avenues of expression and experimentation.
Advantages of Generative AI Systems
Generative AI enables automated content creation and personalization, allowing businesses to scale their creative output and tailor content to individual preferences. From generating personalized product recommendations to creating dynamic advertisements, generative AI revolutionizes content marketing strategies.
In the field of design and art, generative AI serves as a tool for inspiration and collaboration. By generating novel design concepts and exploring unconventional artistic styles, generative AI expands the boundaries of creativity and fosters innovation in visual arts and design.
Challenges and Limitations of Generative AI
One of the challenges associated with generative AI is maintaining quality control and ensuring the authenticity of generated content. As AI algorithms generate increasingly realistic outputs, distinguishing between authentic and AI-generated content becomes more challenging, raising concerns about credibility and trustworthiness.
Generative AI blurs the lines between original and derivative works, posing challenges to traditional notions of intellectual property rights. As AI-generated content becomes more prevalent, questions arise regarding ownership, attribution, and copyright protection in creative industries.
Generative AI algorithms may inherit biases present in training data, leading to unintended outcomes and perpetuating existing inequalities. As AI-generated content influences perceptions and cultural narratives, addressing bias and discrimination becomes essential to promote diversity and inclusion in creative expression.
Key Differences Between Predictive and Generative AI
- Purpose and Functionality: Predictive AI focuses on forecasting future outcomes based on historical data patterns, whereas generative AI is geared towards creating new content or generating novel solutions to problems.
- Data Requirements and Processing Methods: Predictive AI relies on structured datasets and statistical modeling techniques to make predictions, while generative AI utilizes deep learning algorithms and neural networks to generate new content from unstructured data.
- Impact on Industries and Society: Predictive AI influences decision-making processes and business strategies across various industries, whereas generative AI fosters creativity and innovation in artistic and design domains.
- Addressing Bias and Fairness in AI Algorithms: As AI algorithms increasingly influence decision-making processes in various domains, ensuring fairness and mitigating bias becomes paramount to uphold ethical standards and promote social equity. By implementing measures such as bias detection, algorithmic transparency, and fairness-aware training techniques, developers can minimize the impact of biases and ensure that AI systems treat individuals fairly and impartially.
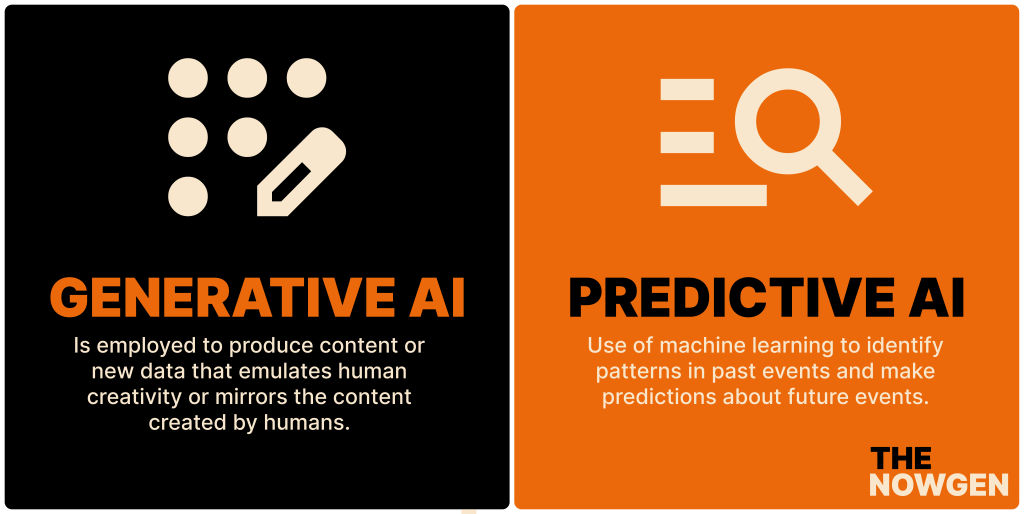
In conclusion, decoding AI trends, particularly predictive AI and generative AI, provides valuable insights into the evolving landscape of artificial intelligence and its profound impact on industries and society. As predictive AI continues to revolutionize decision-making processes and predictive analytics, generative AI unleashes creativity and innovation in content creation and design domains. By understanding the key differences, applications, and challenges of predictive and generative AI, stakeholders can harness the transformative potential of AI technologies while navigating ethical, regulatory, and societal implications to ensure a sustainable and equitable future.